Regulatory Rapporteur
January 2024 | Volume 21 | No.1
Licence notice
Copyright © 2015-2023 The Organisation for Professionals in Regulatory Affairs Ltd. T/A Regulatory Rapporteur − All Rights Reserved. This work is licensed to Bruno Speder for the purposes of academic and personal reasonable use only.
Notwithstanding this licence, no part of materials published in Regulatory Rapporteur may be reproduced without the express written permission of the publisher.
As a general rule, permission should be sought from the rights holder to reproduce any substantial part of a copyrighted work. This includes any text, illustrations, charts, tables, photographs, or other material from previously published sources.
To obtain permission(s) to re-use content published in Regulatory Rapporteur please email publications@topra.org.
To join TOPRA please click here.
Introduction
The recent publication of papers by the European Medicines Agency (EMA)[1] and the Food and Drug Administration (FDA)[2][3] has spurred the debate on the use of artificial intelligence (AI) in drug development and how it can be used to bring novel compounds to the market.
It is currently estimated that there are >1060 (greater than 10 to the power 60) molecules that have the potential to be used as drugs.[4] The current drug discovery process is a time-consuming and expensive endeavour, and can be accelerated by using AI.[5]
Artificial intelligence (AI) can recognise hit and lead compounds and provide a quicker validation of the drug target and optimisation of the drug structure design. With the ever rising cost of drug development, AI allows for a more predictive and data driven approach while enabling to de-risk the development of new drugs. This improves the probability of success in research and development (R&D).
In silico approaches are not new in drug development, in silico modelling has been successfully used by pharma for pharmacokinetic/pharmacodynamic (PK/PD) modelling, statistical modelling, trial design etc in the past decades.[6]
The use of biological modelling however has lagged behind, as the complexity and diversity of biological systems has hindered the development of accurate and reliable models…
The use of biological modelling however has lagged behind, as the complexity and diversity of biological systems has hindered the development of accurate and reliable models. Genetic analysis has been an area of great success, with breakthroughs in cancer treatments and subpopulation analysis to identify patients who will respond to treatment and who should be recruited onto clinical trials. Specialist companies have accumulated vast biobanks of data and samples accompanied by whole genome sequencing which can be used as a fee for service tool by drug developers.
In addition to genetics, modern methods have created a wealth of molecular data and the data-insight gap is growing whereby the exponential growth in data is accelerating at a greater pace than the accompanying insights. A number of companies have sought to close this gap by building tissue specific disease models as a tool to provide A/B testing of drugs in a virtual environment. A simple analogy is to examine the pace at which clinical care is benefitting from the boom in data. Immunology has revealed a vast swathe of information on immune cells, vaccine responses and susceptibility to infection but the clinical insight gained from a complete blood count has not changed dramatically in the past 20 years.
In drug development the challenges lie with implementation including the generation of sufficient high-quality data, incorporation of data and structuring the analysis to provide real insight.
AI approaches in drug discovery
Drug discovery brings a diversity of challenges and a wide range of AI-enabled tools have emerged to reflect this. An overview of the different uses of AI in drug discovery is given in Table 1.[7]
Table 1: Overview uses AI in Drug Discovery (adapted from Sellwood 2018)
AI is intrinsically data driven and therefore it is prone to the same biases that pharma have experienced in recent years such as racial and geographic over representations, as a result of clinical trials which have been focused on western societies. Arguably the question of what data an analysis is based on comes before the actual analysis.[8],[9]
The first step of AI-adoption is identifying what it is you are trying to achieve and building stepwise from that point to ensure the model and underlying data are adequately designed to achieve the end goal.
Integrating AI in the drug discovery process
Drug development is an interdisciplinary activity due to the wide range of expertise required to navigate nonclinical and clinical development.
Approaches to AI integration in discovery programs vary at a company level. Some approaches have limited immersion and strived for unsupervised learning models which have limited exposure to the learnt experience of those working in the field. This independent approach could be more likely to produce revolutionary insight and change but comes with its own challenges.
Controlled interactions between teams can assist in fostering novel approaches and viewpoints. This perspective is counteracted by the operational challenges of AI adoption, whereby biology is nuanced and AI-outputs can be difficult to interpret. Difficulty in knowing how the outcome was arrived at can pose difficulties in validating the outcome in the real world.
Each AI model is influenced by the question which drives its development:
- Can we find a drug that binds to undruggable proteins?
- How will drug X perform in patient Y?
- What targets are most likely to prevent or treat a given disease?
Each requires a different approach and often, different tools. The issues needing consideration vary greatly and require a diligence process that understands the underlying data, the methods, the problem and how the outputs will be structured. Limiting the bias introduced by the underlying datasets and during construction of the AI-model modelling is key.
We understand chemical interactions with a high degree of confidence. Free-energy models can accurately predict how a chemical reaction will play out and this has translated into protein-ligand binding and protein folding models with exceptional success. It is now possible to quickly fold a protein. In 1969, Cyrus Levinthal calculated it would take a typical 300 amino acid protein longer than the existence of the universe to fold were it to try each conformation. But proteins fold near instantaneously and with remarkable precision and consistency.
Computational models have been able to match real world accuracy but still lag when it comes to speed. We have however been remarkably successful in this field where we can confidently predict protein conformations or like the Baker Lab at the University of Washington, we can design completely new proteins to fit any shape. AI companies in these areas have been highly successful. A number of biotech companies have made significant progress in bringing AI-developed compounds towards clinical trials.
AI-models to predict how the body behaves, disease pathology or how drugs behave come with different challenges…
AI-models to predict how the body behaves, disease pathology or how drugs behave come with different challenges. Unlike drug discovery where we know the variables and can define the key parameters such as spatial distribution, free energy and chemical interactions, we do not yet know the key parameters or full set of parameters that define the human body. Proteomics, genomics, transcriptomics, metabolomics, epigenomics, microbiomics, and more, create the complex web called life. How these systems interact is only partially understood and the availability of complete datasets which can track these interactions are limited.
Data is becoming available at an exponential rate and we are in an age where companies are trying to harvest these datasets to enable the next generation of AI models. Drug development relies on a large number of animals and poses intrinsic risk to the health of subjects, AI models offer the potential that we can begin to reduce our reliance on in vivo testing and may in the near future begin to get more regulatory approvals when combined with in vitro methods such as a organoids and 3D cell cultures.
Although the uncertainty increases with complexity, the insight offered by AI models of human disease can provide valuable information during drug discovery. Many drugs fail in humans because they were developed in rodent models and many successful drugs never make it to the clinic because they failed to work in animals. The early stage insight of AI-clinical data during drug development not only de-risks but can provide mechanistic clues that can improve on the models selected during drug development.
In the recent COVID-19 pandemic, AI was used to re-purpose Baricitinib for COVID-19 patients in the UK, which was later validated by the World Health Organization (WHO).[10] AI also helped in optimising COVID-19 vaccines.[11]
AI and small biotech companies
AI tools are an enticing prospect for emerging biotech companies, although they often lack the resources to fully do this inhouse. A wide range of AI companies are developing bespoke models to aid drug development and oftentimes need to commit large resources and skills to contend in a competitive environment. Contingent value right based deals can benefit both partners in a biotech: AI collaboration whereby the AI company gets the insight of working with drug development experienced teams and can tailor their tools to meet those needs, and the biotech company can get unparalleled insights and first mover advantages by leveraging the unique insights on offer from world leading computational models.
Regulatory considerations
As illustrated by the recent publication of reflection and discussion papers by the EMA and the FDA on AI use, there is a growing need for regulatory guidance on the topic.
The regulatory impact is directly related to the phase in the medicinal product lifecycle, the application of AI in the process of (early) drug discovery may be a low risk setting from a regulatory perspective, as non-performance will often lead to the product not being developed further.
In this context it is essential that all models and datasets are assessed for risks of bias and other sources of discrimination of non-majority genotypes and phenotypes, both from a quantitative as data quality perspective.
In cases where impact on regulatory decision-making is high, interaction with regulators is always recommended via Scientific Advice. Early interaction on experimental technology is provided by the EMA Innovation Task Force (ITF).
CASE STUDY: Poolbeg’s influenza AI programme
Poolbeg Pharma has access to unique disease progression data from influenza human challenge trials.
During influenza human challenge studies, healthy volunteers were administered a well-characterised strain of influenza virus. These studies offer a number of distinct advantages[12],[13]
- Known time and dose of infection
- Verifiable clinical assessment
- Multi-parametric dataset – both clinical and biological data collected
- Clean data, as studies are performed under controlled circumstances in a quarantine unit, there is no risk of co-infection with other pathogen
Based on the above, data collected from these challenge trials offer unique insights into the dynamics of influenza disease.
We used a provider who has built world-class validated AI models which can, among other methods, extrapolate immune cell behaviour based on bulk transcriptomics. This resulted in a deep understanding of the mechanism of influenza infection, progression and recovery and, as a result, successfully identified multiple novel drug targets for this disease and new potential drug candidates. Unlike traditional target discovery in which years of lab-based research is performed which may converge on nodes of disease with potential therapeutic potential, AI analysis lends the ability to rapidly perform systems biology-based analysis to identify and prioritise therapeutic targets. Importantly these targets are provided in tandem with associated hypothesis which can be used to design focused validation assays to confirm expected effects in disease models. This provides a rapid and cost-effective tool to validate novel targets for treatment of disease.
In a second step, these targets and candidates will be further assessed by a multi-disciplinary team and evaluated based on clinical benefits, CMC feasibility and regulatory development strategy.
Conclusion
The above-mentioned precedents of the successful application of AI in the drug discovery process are encouraging and we hope that it will accelerate the role of AI in the drug discovery process. The more difficult question is, will the compound produce the same effect in vivo? Drugs are chemical compounds, which act on a biological system, which are much more complex and not yet fully understood. Yet the growing use of AI in the drug development opens the door for the discovery of novel compounds and new hope for patients.
As no AI-discovered drug has received marketing authorisation and it can take over a decade to traverse that journey there is no empirical evidence to compare AI-models and it will take some time to refine the learnings.
References
[1] EMA (2023) Reflection paper on the use of artificial intelligence in the lifecycle of medicines (EMA/CHMP/CVMP/83833/2023)
[2] FDA (2023) Discussion paper Artificial Intelligence in Drug Manufacturing
[3] FDA - Discussion paper Using Artificial Intelligence and Machine Learning in the Development of Drug and Biological Products
[4] Mak K.-K., Pichika M.R. Artificial intelligence in drug development: present status and future prospects. Drug Discovery Today. 2019;24:773–780
[5] Vyas M. Artificial intelligence: the beginning of a new era in pharmacy profession. Asian J. Pharm. 2018;12:72–76.
[6] Musuamba, FT, Skottheim Rusten, I, Lesage, R, et al. Scientific and regulatory evaluation of mechanistic in silico drug and disease models in drug development: Building model credibility. CPT Pharmacometrics Syst Pharmacol. 2021; 10: 804–825
[7] Sellwood M.A. Artificial intelligence in drug discovery. Fut. Sci. 2018;10:2025–2028.
[8] Robinson M.C., Glen R.C., et al. Validating the validation: reanalyzing a large-scale comparison of deep learning and machine learning models for bioactivity prediction. J. Comput.-Aided Mol. Des. 2020;34(7):717–730.
[9] Bender A., Cortés-Ciriano I. Artificial intelligence in drug discovery: what is realistic, what are illusions? Part 1: ways to make an impact, and why we are not there yet. Drug Discov. Today. 2021;26(2):511–524
[10] Stebbing J., Krishnan V., de Bono S., Ottaviani S., Casalini G., Richardson P.J., Monteil V., Lauschke V.M., Mirazimi A., Youhanna S., et al. Mechanism of baricitinib supports artificial intelligence-predicted testing in Covid-19 patients. EMBO Mol. Med. 2020;12(8)
[11] Zhu Z., Shi C., Zhang Z., Liu S., Xu M., Yuan X., Zhang Y., Chen J., Cai H., Lu J., et al. Torchdrug: a powerful and flexible machine learning platform for drug discovery. 2022.
[12] Adams-Phipps et al. A Systematic Review of Human Challenge Trials, Designs, and Safety. Clinical Infectious Diseases (2023)
[13] Baay et al. Human Challenge trials in vaccine development. Biologics (2019)
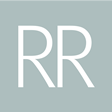
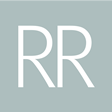
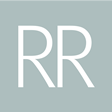